Suggestions
Mayo Oshin
AI advisor & Angel Investor
Mayo Oshin is an accomplished writer who focuses on science-based ideas to assist leaders and organizations in developing better habits and making improved decisions.
His work is highly regarded and has been featured in prominent media outlets like Business Insider, Inc magazine, Entrepreneur magazine, Quartz, and Thrive Global, catering to business owners and executives striving to reach their full potential.
Mayo Oshin has a strong educational background, including a Master of Liberal Arts from Harvard Extension School, a BSc in Economics from the University of Bristol, and an Advanced Diploma in Insurance from the Chartered Institute of Insurance.
He is the Founder and Editor-in-chief of MayoOshin.Com, where he continues to share his insights and knowledge to empower individuals and organizations.
Highlights
One of the major challenges with building AI chat apps and agents powered by RAG is the complexity of creating, storing, and syncing embeddings with real-time source data.
If this isn't managed effectively, your AI app will likely generate outdated, inaccurate information leading users to lose trust and abandon use of the app. Fortunately, there's a simple solution that eliminates this complexity.
pgai Vectorizer by @TimescaleDB helps to ensure that your AI app always utilises relevant, up-to-date data via efficient RAG and vector search processes.
With just one line of SQL (as per image below), you can automate embedding creation and synchronization directly within PostgreSQL. Once you're set up, pgai Vectorizer will automatically sync embeddings with inserts, updates, deletes to source data. No need for you to build external pipelines or synchronization services.
pgai Vectorizer also enables A/B testing of different embedding models and data chunking methods without reprocessing data yourself.
In a nutsell, pgai benefits include:
- Vector embeddings in PostgreSQL: Create, store, and search embeddings all in PostgreSQL.
- No more stale embeddings: Keeps embeddings synced as your underlying data changes.
- All in SQL: Everything is configured in SQL, no extra tools needed.
- Familiar postgreSQL ecosystem: pgai Vectorizer works with other popular tools for AI in PostgreSQL, like the pgvector, pgvectorscale, and pgai extensions.
If you’re looking to simplify your AI infra for your RAG, search or AI agent app, check out the pgai Vectorizer Github repo here: https://t.co/0szormuVFE
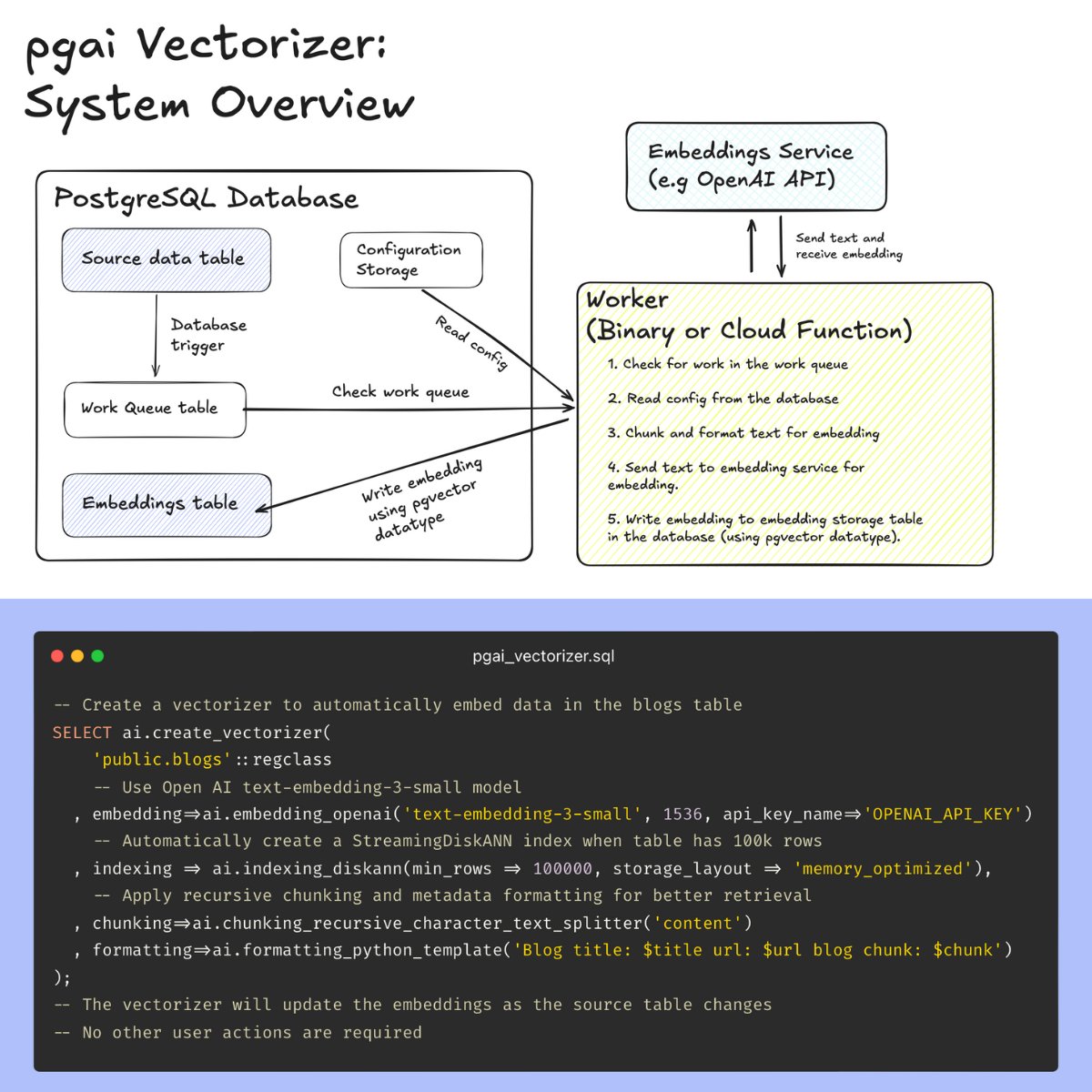